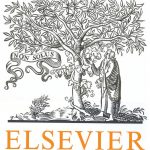
ترجمه مقاله نقش ضروری ارتباطات 6G با چشم انداز صنعت 4.0
- مبلغ: ۸۶,۰۰۰ تومان
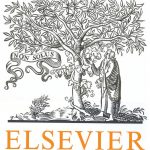
ترجمه مقاله پایداری توسعه شهری، تعدیل ساختار صنعتی و کارایی کاربری زمین
- مبلغ: ۹۱,۰۰۰ تومان
Abstract
Accurate knowledge of the vehicle states is the foundation of vehicle motion control. However, in real implementations, sensory signals are always corrupted by delays and noises. Network induced time-varying delays and measurement noises can be a hazard in the active safety of over-actuated electric vehicles (EVs). In this paper, a brain-inspired proprioceptive system based on state-of-the-art deep learning and data fusion technique is proposed to solve this problem in autonomous four-wheel actuated EVs. A deep recurrent neural network (RNN) is trained by the noisy and delayed measurement signals to make accurate predictions of the vehicle motion states. Then unscented Kalman predictor, which is the adaption of unscented Kalman filter in time-varying-delay situations, combines the predictions of the RNN and corrupted sensory signals to provide better perceptions of the locomotion. Simulations with a high-fidelity, CarSim, full-vehicle model are carried out to show the effectiveness of our RNN framework and the entire proprioceptive system.
VI. CONCLUSION
A brain-inspired method, which combines DL and data fusion was proposed to overcome the feedback delays and noises in autonomous FWIA s EVs in this paper. The simulation results demonstrate that modern RNN architecture and learning method can successfully extract nonlinear dynamic models of the vehicle from sensory data, and UKP can fuse the two streams of information well. The performance of yaw rate tracking of FWIA EVs can be improved with the introduction of our proprioceptive system.
In this paper, the training was conducted offline. However, autonomous vehicles face an ever-changing environment in operation. Some parameters of vehicles, like mass, friction coefficient are not constant, and the dynamic models of vehicle motion are time-varying. As such, the online learning method which can adapt to the changes in the environment along with the learning, inference, and selection of multimodels are our goals for the future study. Besides, the delays in the feedforward loop are ignored in this paper. Although these delays are not as obvious as those in the feedback loop, they still exist and can affect the closed loop control performance. The real-time performance considers feedback delay, feedforward delay, and VCU computational delay should be carefully studied in the future.