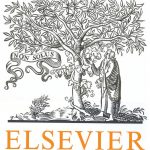
ترجمه مقاله نقش ضروری ارتباطات 6G با چشم انداز صنعت 4.0
- مبلغ: ۸۶,۰۰۰ تومان
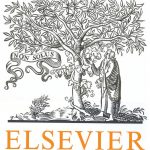
ترجمه مقاله پایداری توسعه شهری، تعدیل ساختار صنعتی و کارایی کاربری زمین
- مبلغ: ۹۱,۰۰۰ تومان
In mining data streams the most popular tool is the Hoeffding tree algorithm. It uses the Hoeffding’s bound to determine the smallest number of examples needed at a node to select a splitting attribute. In the literature the same Hoeffding’s bound was used for any evaluation function (heuristic measure), e.g., information gain or Gini index. In this paper, it is shown that the Hoeffding’s inequality is not appropriate to solve the underlying problem. We prove two theorems presenting the McDiarmid’s bound for both the information gain, used in ID3 algorithm, and for Gini index, used in Classification and Regression Trees (CART) algorithm. The results of the paper guarantee that a decision tree learning system, applied to data streams and based on the McDiarmid’s bound, has the property that its output is nearly identical to that of a conventional learner. The results of the paper have a great impact on the state of the art of mining data streams and various developed so far methods and algorithms should be reconsidered.
در جریان های داده کاوی محبوب ترین ابزار، الگوریتم درخت Hoeffding می باشد. از کران Hoeffding برای تعیین کوچکترین تعداد نمونه های مورد نیاز در یک گره که برای انتخاب ویژگی تقسیم استفاده می کند.در ادبیات کران Hoeffding مشابهی برای هر تابع ارزیابی (اندازه گیری اکتشافی)، به عنوان مثال، بدست آوری اطلاعات و یا شاخص Gini مورد استفاده قرار می گرفت. در این مقاله، نشان داده شده است که نابرابری Hoeffding برای حل مشکل اساسی مناسب نیست. ما دو قضیه را اثبات می کنیم که کران McDiarmid را برای هر دو اطلاعات مورد استفاده در الگوریتم3 ID، و شاخص Gini ، در طبقه بندی و الگوریتم درختان رگرسیون (CART) مورد استفاده قرار می دهد. نتایج مقاله تضمین می کند که یک سیستم یادگیری درخت تصمیم گیری، اعمال شده به جریان های داده ای و بر اساس کران McDiarmid ، دارای ویژگی است که در آن خروجی تقریبا یکسان با یادگیرنده معمولی است. نتایج مقاله تاثیر به سزایی بر روی وضعیت جریان های داده ای و روش های مختلف توسعه یافته تا کنون دارد و الگوریتم ها باید تجدید نظر گردند.