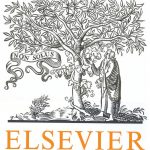
ترجمه مقاله نقش ضروری ارتباطات 6G با چشم انداز صنعت 4.0
- مبلغ: ۸۶,۰۰۰ تومان
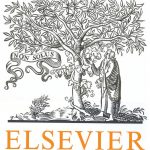
ترجمه مقاله پایداری توسعه شهری، تعدیل ساختار صنعتی و کارایی کاربری زمین
- مبلغ: ۹۱,۰۰۰ تومان
Abstract
Visual attributes, from simple objects (e.g., backpacks, hats) to soft-biometrics (e.g., gender, height, clothing) have proven to be a powerful representational approach for many applications such as image description and human identification. In this paper, we introduce a novel method to combine the advantages of both multi-task and curriculum learning in a visual attribute classification framework. Individual tasks are grouped after performing hierarchical clustering based on their correlation. The clusters of tasks are learned in a curriculum learning setup by transferring knowledge between clusters. The learning process within each cluster is performed in a multi-task classification setup. By leveraging the acquired knowledge, we speed-up the process and improve performance. We demonstrate the effectiveness of our method via ablation studies and a detailed analysis of the covariates, on a variety of publicly available datasets of humans standing with their full-body visible. Extensive experimentation has proven that the proposed approach boosts the performance by 4%–10%.
1. Introduction
Vision as reception. Vision as reflection. Vision as projection. –Bill Viola, note 1986
When we are interested in providing a description of an object or a human, we tend to use visual attributes to accomplish this task. For example, a laptop can have a wide screen, a silver color, and a brand logo, whereas a human can be tall, female, wearing a blue t-shirt and carrying a backpack. Visual attributes in computer vision are equivalent to the adjectives in our speech. We rely on visual attributes since (i) they enhance our understanding by creating an image in our head of what this object or human looks like; (ii) they narrow down the possible related results when we want to search for a product online or when we want to provide a suspect description; (iii) they can be composed in different ways to create descriptions; (iv) they generalize well as with some finetuning they can be applied to recognize objects for different tasks; and (v) they are a meaningful semantic representation of objects or humans that can be understood by both computers and humans. However, effectively predicting the corresponding visual attributes of a human given an image remains a challenging task [1]. In reallife scenarios, images might be of low-resolution, humans might be partially occluded in cluttered scenes, or there might be significant pose variations.
6. Conclusion
Given a set of tasks that need to be learned we sought to find an answer to how we can learn them effectively and what would be the optimal way in terms of performance, speed, and simplicity. Learning each task separately, although very simple, lacks in terms of performance since it does not exploit the information from other tasks. Learning all tasks at the same time in a multitask classification scenario is relatively fast, easy to implement, and employs knowledge from other tasks to boost the classification performance. Curriculum learning is a learning scheme in which samples or tasks are not treated as equally easy or hard, but are instead presented to the model in a meaningful way so as to increase generalization and performance. Since learning a large number of tasks one at a time is computationally expensive, we opted for learning clusters of tasks in a curriculum. In each cluster of visual attributes, we proposed to learn the corresponding tasks in a multi-task classification setup.
نمادهای دیداری – از یک شی (مثل کوله پشتی و یا کلاه) تا ویژگی های زیستی نرم (مثل جنسیت، طول و پوشش) می توانند روش های نمایشی قوی در کاربردهای مختلف مثل توصیف تصویر و شناسایی انسان باشند. در این تحقیق، ما روش جدیدی را برای ترکیب مزایای یادگیری چند تمرینی و برنامه ای در چارچوب دسته بندی نمادهای دیداری معرفی کرده ایم. وظایف فردی بعد از اجرای دسته بندی هرمی و بر اساس همبستگی ها گروه بندی می شوند. دسته بندی تکالیق در یک موقعیت برنامه ریزی یادگیری و از طریق انتقال دانش بین دو دسته ها انجام می شود. با افزایش دانش کسب شده، فرآیند و عملکرد یادگیری را می توان ارتقا داد. ما در این تحقیق، کارایی روش مطرح شده را از طریق تحقیقات قبلی و تحلیل کامل کو واریانس برای مجموعه اطلاعات مختلف انسانی قابل مشاهده بررسی کرده ایم. تجارب گسترده اثبات کرده اند که روش مطرح شده از لحاظ عملکرد تا حدود 4 تا 10 درصد افزایش می یابد.
1. مقدمه
نگرش به عنوان واکنش ، نگرش به عنوان انعکاس، نگرش به عنوان تجسم بیل ویولا -1986
وقتی که علاقه مند به ارائه توصیف یک جسم یا انسان باشیم، باید از نمادهای دیداری برای رسیدن به این هدف استفاده کنیم. برای مثال، یک لپ تاپ می تواند دارای صفحه نمایش پهن، رنگ نقراه ای و علامت برند باشد، در حالی که یک فرد می تواند قد بلند، زن ، دارای تی شرت آبی و کوله پشتی توصیف شود. نمادهای دیداری در منظره کامپیوتری معادل صفت در گفتار هستند. تاکید ما بر نمادهای دیداری می باشد زیرا 1- درک ما را از طریق ایجاد یک تصویر در ذهن و شبیه آنچه دیده می شود افزایش می دهد 2- نتایج احتمالی را در صورت تمایل برای جستجوی آنلاین یک محصول محدود سازند 3- می توانند به شیوه های مختلف برای توصیف ترکیب شوند 4- به خوبی می توانند تعمیم داده شوند و برای تشخیص اشیا از تمرین های مختلف استفاده شوند 5- نمایش معناداری از اشیا و انسان ها هستند و می توانند توسط کامپیوتر و انسان درک شوند. به هر حال، پیش بینی دقیق و موثر نمادهای دیداری مرتبط با تصویر داده شده از انسان می تواند یک کار چالش برانگیز باشد (1). در سناریوهای واقعی، تصاویر و عکس ها می توانند دقت پایین داشته باشند و انسان ها می توانند در صحنه های مات نمایش داده شوند.
6.نتیجه گیری
با ارائه مجموعه ای از تکالیفی که باید آموزش داده شوند به دنبال پاسخ دادن به این سوال هستیم که چگونه می توانیم این تکالیف را به صورت موثر یاد بگیریم و بهترین روشهای بادگیری از نظر عملکرد، سرعت و سهولت کدام است. یادگیری هر کدام از تکالیف به صورت جداگانه حتی در صورت ساده بودن فاقد معیار عملکرد است زیرا در آن اطلاعات حاصل از سایر تکالیف در نظر گرفته نشده است. یادگیری تمام تکالیف در یک زمان و در سناریوهای دسته بندی چند تمرینی کاری سریع و قابل اجرا است و در آن از دانش و معلومات سایر تکالیف برای تقویت عملکرد دسته بندی استفاده می شود. یادگیری برنامه ای یک نوع طرح آموزشی است که در آن تکالیف یا نمونه های دارای درجه آسان یا سخت در نظر گرفته نمی شوند، بلکه به صورت معنادار در مدل ارائه می شوند تا عملکرد و قابلیت تعمیم پذیری آن افزایش یابد. چون یادگیری تکالیف متعدد در یک زمان از نظر محاسبه پر هزینه می باشد، دسته بندی تکالیف را در برنامه درسی انجام دادیم. در هر کدام از دسته های نمادهای دیداری، بیان می کنیم که یادگیری تکالیف مرتبط در موقعیت وچود چند تمرین اتفاق می افتد.