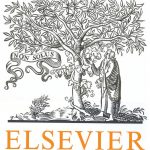
ترجمه مقاله نقش ضروری ارتباطات 6G با چشم انداز صنعت 4.0
- مبلغ: ۸۶,۰۰۰ تومان
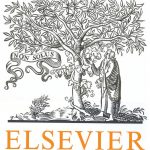
ترجمه مقاله پایداری توسعه شهری، تعدیل ساختار صنعتی و کارایی کاربری زمین
- مبلغ: ۹۱,۰۰۰ تومان
Abstract
The authors developed a forecasting model for Luxembourg, able to predict the expected regional PV power up to 72 hours ahead. The model works with solar irradiance forecasts, based on numerical weather predictions in hourly resolution. Using a set of physical equations, the algorithm is able to predict the expected hourly power production for PV systems in Luxembourg, as well as for a set of 23 chosen PV-systems which are used as reference systems. Comparing the calculated forecasts for the 23 reference systems to their measured power over a period of 2 years, revealed a comparably high accuracy of the forecast. The mean deviation (bias) of the forecast was 1.1% of the nominal power – a relatively low bias indicating low systemic error. The root mean square error (RMSE), lies around 7.4% - a low value for single site forecasts. Two approaches were tested in order to adapt the short-term forecast, based on the present forecast deviations for the reference systems. Thereby, it was possible to improve the very short term forecast on the time horizon of 1-3 hours ahead, specifically for the remaining bias, but also systemic deviations can be identified and partially corrected (e.g. snow cover).
Conclusions and Outlook
Finally, the performance of the individual hourly power forecasts for the 23 reference systems, evaluated over a period of 2 years, is already quite promising. Without any adaptations of the forecast, based on the measurements of the reference systems, the mean deviation (bias) of the forecast was 1.1% of the nominal power (biasdt = 2.2%) – indicating low systemic error. Also the overall mean RMSE of 7.4% (RMSEdt = 10.0%) indicates a low dispersion of the power forecast. A huge collection of performance indicators for different forecast schemes can be found in recent review papers, such as [1] and [8], but a direct comparison is difficult. As Antonanzas stated [1], besides the large set of different indicators used and lack standardisation in their calculation, there are many factors which hamper a comparison: Climate conditions, day- and night-time values used, base of normalisation, sample aggregation, spatial aggregation level and testing period. Generally, it has been found that, by far, the main uncertainties arise from the irradiance forecast, which is not surprising, but nevertheless the accuracy of the technical part of the model is very satisfactory.