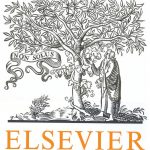
ترجمه مقاله نقش ضروری ارتباطات 6G با چشم انداز صنعت 4.0
- مبلغ: ۸۶,۰۰۰ تومان
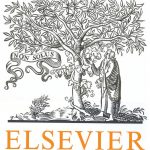
ترجمه مقاله پایداری توسعه شهری، تعدیل ساختار صنعتی و کارایی کاربری زمین
- مبلغ: ۹۱,۰۰۰ تومان
Abstract
In view of the two problems of the SVM algorithm in processing large data, the paper proposed a weighted Euclidean distance, radial integral kernel function SVM and dimensionality reduction algorithm for large data packet classification. The SVM cannot handle multi classification and time of building model is long. The algorithm solved these problems. The improved algorithm reconstructs the data feature space, makes the boundary of different data samples clearer, shortens the modeling time, and improves the accuracy of classification. The proposed method verified the feasibility and effectiveness with experiments. The experimental results show that the improved algorithm can achieve better results when multi-duplicated samples and large data capacity are used for multi classification.
CONCLUSION
In the paper, a weighted Euclidean distance, the radial product kernel function and SVM algorithm are constructed to solve the problem of large data feature extraction and big data classification. Effective reduction the data sample of establishing the test model through data filtering preprocessing. The number of support vector samples established the test model has decreased in varying degrees. The overall detection accuracy reached the highest after selecting and weighting. It is shown that the selection algorithm can effectively remove the nonsignificant interference samples and improve the generalization of the model. The results show that the class distribution of the weighted samples is more obvious. The training time is significantly shorter. The detection effect is further improved after weighting.