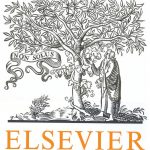
ترجمه مقاله نقش ضروری ارتباطات 6G با چشم انداز صنعت 4.0
- مبلغ: ۸۶,۰۰۰ تومان
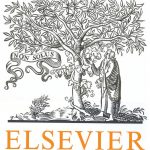
ترجمه مقاله پایداری توسعه شهری، تعدیل ساختار صنعتی و کارایی کاربری زمین
- مبلغ: ۹۱,۰۰۰ تومان
Abstract
Received Signal Strength Indicator (RSSI) is affected significantly by multi-path fading, building structure and obstacles in indoor environments, which lead to similar fingerprints problem and noise. To improve the performance of traditional fingerprinting method, the measurements provided by inertial sensors can be leveraged. Particle filter (PF) method is a widely chosen algorithm for sensor fusion. However, the initialization and weighting process are problematic in indoor positioning systems. This paper proposes a new PF scheme which yield a smooth and stable localization experience. To differentiate similar fingerprints, a single-hidden layer feed-forward networks (SLFNs) is used to model the multiple probabilistic estimations and improve the performance of the PF. Meanwhile, a new initialization algorithm using Random Sample Consensus (RANSAC) is presented to reduce the convergence time. Experimental measurements were carried out to determine the performance of the proposed algorithm. The results indicate that the positioning error of proposed scheme falls to less than 1.2 m which is better than the error reported in comparable approaches.
6. Conclusion
In this paper, a new particle filter with a hardware-free initialization phase is presented to improve the accuracy of indoor location positioning using received signal strength. The hardwarefree initialization is implemented by RANSAC algorithm. This algorithm filters out outliers from the fingerprinting estimations by a constructed PDR model. Inliers are remained to acquire the initial point and the current location. The PF is initializing based on the current location. This initialization phase achieves 1.1 (m) average error distance in the experimental demonstration. For enhancing the fusion of fingerprinting and PDR, we proposed a SFLNs based model fitting algorithm. The algorithm takes advantage of the probabilities of all the reference points from fingerprinting method. The algorithm fits a SFLNs model to the probabilities and constructs a probability surface over the interested area. The particles are weighted by this continuous surface to reduce the error. This approach makes sure that the particles would not suffer from the similar fingerprints issue. The experimental results show about 1.2 (m) average error distance in compare to 2.2 (m) in comparative methods.