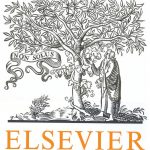
ترجمه مقاله نقش ضروری ارتباطات 6G با چشم انداز صنعت 4.0
- مبلغ: ۸۶,۰۰۰ تومان
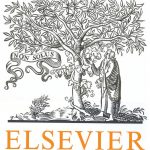
ترجمه مقاله پایداری توسعه شهری، تعدیل ساختار صنعتی و کارایی کاربری زمین
- مبلغ: ۹۱,۰۰۰ تومان
abstract
This paper summarizes, critiques, and synthesizes the time-series literature in accounting pertaining to quarterly accounting numbers. It reviews work on quarterly earnings, quarterly balance sheet and income statement subcomponents, and quarterly cash-flows from operations (CFOs). Several salient findings emerge. First, the premier ARIMA models attributed to Foster (1977), Griffin (1977) and Brown and Rozeff (1979) were identified on relatively small samples dominated by “old economy” firms. It appears that the descriptive validity of these ARIMA structures must be called into question when analyzing more current databases replete with high-technology, regulated, and financial-service firms (Lorek & Willinger, 2007). Second, the use of ARIMA-based analytical review procedures in audit settings is not cost effective. Third, recent evidence (Lorek & Willinger, 2008, 2011) supports the univariate Brown & Rozeff (100) × (011) ARIMA model as the best statistically-based prediction model for quarterly CFO, a finding of considerable import to analysts, investors, and researchers.
5. Quarterly CFO
Bowen, Burgstahler, and Daley (1986) argue that analysts, retail investors, and accounting researchers are interested in cash-flow forecasting. This interest stems from a diverse set of decision settings for which CFO predictions serve as inputs including: risk assessment, the accuracy of credit-rating predictions, and firm valuations using discounted cash flows. Barniv, Myring, and Thomas (2005), however, document the current unavailability of analysts' multi-step ahead quarterly CFO forecasts. Yet, financial statement analysis texts underscore the need for long-term CFO forecasts for firm valuations (Palepu & Healy, 2013). Therefore, research on statistically-based quarterly CFO models takes on added importance in this setting. Hopwood and McKeown (1992) used an algorithm which added back depreciation and amortization charges on a quarterly basis to earnings in order to derive a proxy for quarterly CFO. Their sampling filters resulted in a relatively small sample of manufacturing firms (n = 60) with a complete time series of quarterly CFO from 1976 to 1987. The examination of SACFs revealed that the quarterly CFO series exhibited substantially lower levels of autocorrelation than their corresponding quarterly EPS series. Using the premier quarterly EPS seasonal ARIMA models, they provided predictive results that quarterly CFO was significantly more difficult to predict than quarterly EPS, consistent with the descriptive evidence on autocorrelation. Hopwood and McKeown called for future research to identify idiosyncratic prediction models for quarterly CFO with potentially unique model structures perhaps different from the premier models for quarterly EPS.