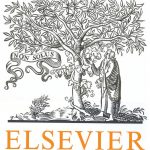
ترجمه مقاله نقش ضروری ارتباطات 6G با چشم انداز صنعت 4.0
- مبلغ: ۸۶,۰۰۰ تومان
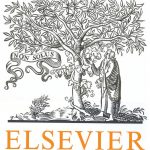
ترجمه مقاله پایداری توسعه شهری، تعدیل ساختار صنعتی و کارایی کاربری زمین
- مبلغ: ۹۱,۰۰۰ تومان
Due to the complexity of predicting future earthquakes, machine learning algorithms have been used by several researchers to increase the Accuracy of the forecast. However, the concentration of previous studies has chiefly been on the temporal rather than spatial parameters. Additionally, the less correlated variables were typically eliminated in the feature analysis and did not enter the model. This study introduces and investigates the effect of spatial parameters on four ML algorithms' performance for predicting the magnitude of future earthquakes in Iran as one of the most earthquake-prone countries in the world. We compared the performances of conventional methods of Support Vector Machine (SVM), Decision Tree (DT), and a Shallow Neural Network (SNN) with the contemporary Deep Neural Network (DNN) method for predicting the magnitude of the biggest upcoming earthquake in the next week. Information Gain analysis, Accuracy, Sensitivity, Positive Predictive Value, Negative Predictive Value, and Specificity measures were exploited to investigate the outcome of using a new parameter, called Fault Density, calculated using Kernel Density Estimation and Bivariate Moran's I, on the performance of the earthquake prediction, in comparison to other commonly used parameters. We discussed the behavior of the four models while dealing with different combinations of parameters and different classes of earthquake magnitudes. The results showed promising performance of the proposed parameter for the earthquakes of high magnitudes, especially using SVM and DNN models.
1. Introduction
Earthquake is a destructive natural disaster that occurs almost without any warning in advance. It inflicts plenty of casualties and financial loss to human societies. Besides, it can impose several environmental side effects such as surface fault rupture [1] and soil liquefication [2] or initiates other types of disasters like tsunamis [3], landslide [4], and fires [5]. Due to the high potential of destruction and death [6,7] as well as the direct and indirect effects of earthquakes [8], researchers have been vigorously working on the idea of proposing different approaches for earthquake prediction [9–11]. Timely and reliable forecasting can provide the possibility to consider preventive measures for mitigating the devastating effects of powerful earthquakes. Besides, such a forecast would be able to increase the level of public preparedness. A successful forecast determines the geographical location, the time, and the magnitude of an earthquake [12]. Such predictions can save many lives and vast amounts of resources. However, despite proposing various methods using different input parameters, such successful forecasts are rare amongst the past research [13].
به دلیل وجود پیچیدگی هایی در پیشبینی زلزلههای آینده، الگوریتم های یادگیری ماشینی توسط چندین محقق برای افزایش در دقت پیشبینی مورد استفاده قرار گرفته است. علاوه بر این، متغیرهایی هم با همبستگی کمتر به طور معمول در تحلیل ویژگی حذف شده و وارد مدل نشدند. در کل این مطالعه به معرفی و بررسی اثر پارامترهای فضایی بر عملکرد چهار الگوریتم ML برای پیشبینی بزرگی در زلزلههای آینده در ایران به عنوان یکی از کشورهای زلزلهخیز جهان میپردازد. ما عملکرد روشهای معمول مانند، بردار پشتیبان ماشین (SVM)، درخت تصمیم (DT)و شبکه عصبی کم عمق (SNN)را با روش شبکه عصبی عمیق جدید (DNN)برای پیشبینی بزرگای بزرگترین زلزله در هفته آینده مقایسه کردیم. تحلیل اطلاعات، دقت، حساسیت، مقدار اخباری مثبت، مقدار اخباری منفی، و معیارهای ویژگی برای بررسی نتیجه استفاده از یک پارامتر جدید، به نام چگالی خطا، محاسبه شده با استفاده از تخمین چگالی کرنل و واریانس دو متغیره موران I، بر روی عملکرد پیشبینی زلزله، در مقایسه با دیگر پارامترهای معمول مورد استفاده، مورد استفاده قرار گرفتند. ما همچنین رفتار این چهار مدل را در هنگام برخورد با ترکیبهای مختلف پارامترها و طبقات مختلف بزرگی زلزله مورد بحث قرار دادیم. نتایج نشاندهنده عملکرد امیدوارکننده پارامتر پیشنهادی برای زلزلههای با بزرگی بالا، به خصوص با استفاده از روشهای مرسوم بردار پشتیبان ماشین SVM و شبکه عصبی عمیق جدید DNN است.
۱. مقدمه
زلزله همواره یک فاجعه طبیعی مخرب است که تقریبا بدون هیچ هشداری قبلی رخ میدهد. این امر تلفات و خسارات مالی زیادی را به جوامع انسانی وارد میکند. علاوه بر این، میتواند اثرات جانبی زیستمحیطی متعددی از قبیل گسیختگی سطحی گسل و روان گرایی خاک و یا انواع دیگری از بلایا مانند سونامی ، لغزش زمین و آتشسوزی را تحمیل کند. با توجه به پتانسیل بالای تخریب و مرگ زلزله و همچنین اثرات مستقیم و غیر مستقیم زلزله، محققان به شدت بر روی ایده پیشنهادی روشهای مختلف برای پیشبینی زلزله کار کردهاند. پیشبینی به موقع و قابلاعتماد میتواند امکان در نظر گرفتن اقدامات پیشگیرانه برای کاهش اثرات مخرب زلزلههای قدرتمند را فراهم کند. علاوه بر این، چنین پیشبینی میتواند سطح آمادگی عمومی را افزایش دهد. یک پیشبینی موفق همواره موقعیت جغرافیایی، زمان و بزرگی زلزله را تعیین میکند [ ۱۲ ]. چنین پیشبینیهای دقیقی میتواند جان بسیاری از انسان ها و نیز مقدار زیادی از منابع را نجات دهد. با این حال، علیرغم پیشنهاد روشهای مختلف با استفاده از پارامترهای ورودی مختلف، چنین پیشبینیهای موفقی در میان تحقیقات گذشته بسیار کمیاب است [ ۱۳ ].