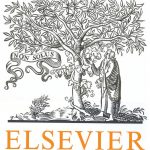
ترجمه مقاله نقش ضروری ارتباطات 6G با چشم انداز صنعت 4.0
- مبلغ: ۸۶,۰۰۰ تومان
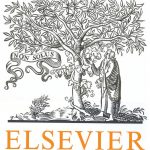
ترجمه مقاله پایداری توسعه شهری، تعدیل ساختار صنعتی و کارایی کاربری زمین
- مبلغ: ۹۱,۰۰۰ تومان
The problem of multi-label classification has attracted great interest in the last decade, where each instance can be assigned with a set of multiple class labels simultaneously. It has a wide variety of real-world applications, e.g., automatic image annotations and gene function analysis. Current research on multi-label classification focuses on supervised settings which assume existence of large amounts of labeled training data. However, in many applications, the labeling of multi-labeled data is extremely expensive and time-consuming, while there are often abundant unlabeled data available. In this paper, we study the problem of transductive multi-label learning and propose a novel solution, called TRAM, to effectively assign a set of multiple labels to each instance. Different from supervised multi-label learning methods, we estimate the label sets of the unlabeled instances effectively by utilizing the information from both labeled and unlabeled data. We first formulate the transductive multi-label learning as an optimization problem of estimating label concept compositions. Then we derive a closed-form solution to this optimization problem and propose an effective algorithm to assign label sets to the unlabeled instances. Empirical studies on several real-world multi-label learning tasks demonstrate that our TRAM method can effectively boost the performance of multilabel classification by using both labeled and unlabeled data.