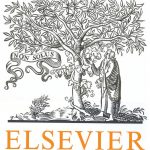
ترجمه مقاله نقش ضروری ارتباطات 6G با چشم انداز صنعت 4.0
- مبلغ: ۸۶,۰۰۰ تومان
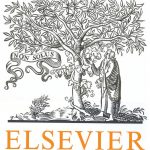
ترجمه مقاله پایداری توسعه شهری، تعدیل ساختار صنعتی و کارایی کاربری زمین
- مبلغ: ۹۱,۰۰۰ تومان
abstract
We study with numerical simulation the possible limit behaviors of synchronous discrete-time deterministic recurrent neural networks composed of N binary neurons as a function of a network’s level of dilution and asymmetry. The network dilution measures the fraction of neuron couples that are connected, and the network asymmetry measures to what extent the underlying connectivity matrix is asymmetric. For each given neural network, we study the dynamical evolution of all the different initial conditions, thus characterizing the full dynamical landscape without imposing any learning rule. Because of the deterministic dynamics, each trajectory converges to an attractor, that can be either a fixed point or a limit cycle. These attractors form the set of all the possible limit behaviors of the neural network. For each network we then determine the convergence times, the limit cycles’ length, the number of attractors, and the sizes of the attractors’ basin. We show that there are two network structures that maximize the number of possible limit behaviors. The first optimal network structure is fully-connected and symmetric. On the contrary, the second optimal network structure is highly sparse and asymmetric. The latter optimal is similar to what observed in different biological neuronal circuits. These observations lead us to hypothesize that independently from any given learning model, an efficient and effective biologic network that stores a number of limit behaviors close to its maximum capacity tends to develop a connectivity structure similar to one of the optimal networks we found.
5. Conclusion
From our exploration of the role of connectivity and symmetry in recursive neural networks, we find two regions that optimize limit behavior storage and signal-response association. The first region is composed of asymmetric/diluted networks, and the second region is formed of symmetric/fully-connected networks. Furthermore, we found a third region made of asymmetric/fullyconnected networks characterized by chaotic and glassy limit behaviors. From these results we are left with the question of why adaptation and evolution selected the first region. Is this because more non-zero elements in the connectivity matrix corresponds to more costly connections? Is it because fully-connected networks imply the existence of two axons between any two neurons and this would be spatially impossible if not technically implausible for large network sizes N? Is it because the natural learning rules that guide neural networks development force them to dynamically evolve in the second region?
Lastly, to partly overcome the smallness of N, we have also analyzed the scaling properties of the main measured quantities. We have found that the scaling behavior for the averaged number of length 1 cycles in fully-connected symmetric networks are perfectly in agreement with theoretical values found by Tanaka and Edwards (1980). Thus the averaged number of length 1 cycles is not biased by the finite size effects. In addition, in the analyzed range for small N, all the scaling laws appear highly robust. These observations do not guarantee that the same occurs for the scaling laws of other observable quantities in diluted networks. Nevertheless, it gives us confidence in the extrapolation of the scaling laws observed in this paper.