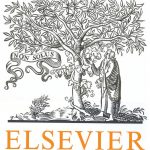
ترجمه مقاله نقش ضروری ارتباطات 6G با چشم انداز صنعت 4.0
- مبلغ: ۸۶,۰۰۰ تومان
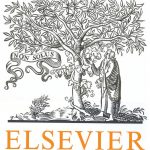
ترجمه مقاله پایداری توسعه شهری، تعدیل ساختار صنعتی و کارایی کاربری زمین
- مبلغ: ۹۱,۰۰۰ تومان
abstract
In this paper we propose a model retraining method for learning more efficient convolutional representations for Content Based Image Retrieval. We employ a deep CNN model to obtain the feature representations from the activations of the convolutional layers using max-pooling, and subsequently we adapt and retrain the network, in order to produce more efficient compact image descriptors, which improve both the retrieval performance and the memory requirements, relying on the available information. Our method suggests three basic model retraining approaches. That is, the Fully Unsupervised Retraining, if no information except from the dataset itself is available, the Retraining with Relevance Information, if the labels of the training dataset are available, and the Relevance Feedback based Retraining, if feedback from users is available. The experimental evaluation on three publicly available image retrieval datasets indicates the effectiveness of the proposed method in learning more efficient representations for the retrieval task, outperforming other CNN-based retrieval techniques, as well as conventional hand-crafted feature-based approaches in all the used datasets.
5. Conclusions
In this paper we proposed a model retraining methodology for enhancing the deep convolutional representations in the retrieval domain. The proposed method suggests three retraining approaches relying on the available information. Thus, if no information is available, the Fully Unsupervised retraining approach is proposed, if the labels are available the Retraining with Relevance Information, and finally if users’ feedback is available the Relevance Feedback based retraining is proposed. We utilize a deep CNN model to obtain the convolutional representations and build the target representations according to each approach, and then we retrain appropriately the network’s weights. We also proposed a combinatory retraining strategy, where the FU retraining approach can be utilized as a pretraining step in order to boost the performance of the RRI and RF approaches. We note that all the proposed approaches are applicable to the fully connected layers too, as well as to other CNN architectures. We should also note that the proposed methodology is applicable to any other CNN-based image retrieval method that utilizes a CNN model to directly extract feature representations. Experimental results indicate the effectiveness of our method, with superior performance over the state of the art approaches, either via a single retraining approach, or by utilizing successive retraining processes.