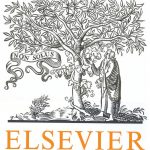
ترجمه مقاله نقش ضروری ارتباطات 6G با چشم انداز صنعت 4.0
- مبلغ: ۸۶,۰۰۰ تومان
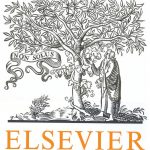
ترجمه مقاله پایداری توسعه شهری، تعدیل ساختار صنعتی و کارایی کاربری زمین
- مبلغ: ۹۱,۰۰۰ تومان
abstract
Learning curves of simple perceptron were derived here. The learning curve of the perceptron learning with noisy teacher was shown to be non-monotonic, which has never appeared even though the learning curves have been analyzed for half a century. In this paper, we showed how this phenomenon occurs by analyzing the asymptotic property of the perceptron learning using a method in systems science, that is, calculating the eigenvalues of the system matrix and the corresponding eigenvectors. We also analyzed the AdaTron learning and the Hebbian learning in the same way and found that the learning curve of the AdaTron learning is non-monotonic whereas that of the Hebbian learning is monotonic.
6. Conclusions
In this paper, we analyzed convergence properties of the perceptron learning, the AdaTron learning and the Hebbian learning, when the teacher was noisy. The learning curves in these cases were analytically derived using a statistical mechanical method and were consistent with the experimental results in our simulation. Our analyses showed that the learning curves of the perceptron learning and the AdaTron learning have an overshoot, that is, the covariance coefficient R of the teacher and the student exceeds the convergence value once. However, the Hebbian learning does not have this property. We showed that these phenomena result from the difference of the eigenvalues and eigenvectors of the system matrix using the asymptotic analysis of dynamical systems. This result may give a method for controlling the learning coefficient η to achieve a faster convergence speed and a lower residual error in the future.