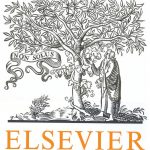
ترجمه مقاله نقش ضروری ارتباطات 6G با چشم انداز صنعت 4.0
- مبلغ: ۸۶,۰۰۰ تومان
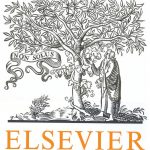
ترجمه مقاله پایداری توسعه شهری، تعدیل ساختار صنعتی و کارایی کاربری زمین
- مبلغ: ۹۱,۰۰۰ تومان
Principal Component Analysis (PCA) is a classical multivariate statistical algorithm for data analysis. Its goal is to extract principal features or properties from data, and to represent them as a set of new orthogonal variables called principal components. Although PCA has obtained extensive successes across almost all the scientific disciplines, it is clear that PCA cannot incorporate the supervised information such as class labels. In order to overcome this limitation, we present a novel methodology to combine the supervised information with PCA by discriminatively selecting the components. Our method use the fisher criterion to evaluate the discriminative abilities of bases of original PCA and find the first n best ones to yield the new PCA projections. Clearly, the proposed method is general to all PCA family algorithms and even can be applied to other unsupervised multivariate statistical algorithms. Furthermore, another desirable advantage of our method is that it doesn’t break the original structure of the PCA components and thereby keeps their visual interpretability. As two examples, we apply our method to incorporate the supervise information with PCA and Robust Sparse PCA (RSPCA) to improve their discriminative abilities. Experimental results on two popular databases demonstrate the effectiveness of our method.