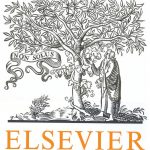
ترجمه مقاله نقش ضروری ارتباطات 6G با چشم انداز صنعت 4.0
- مبلغ: ۸۶,۰۰۰ تومان
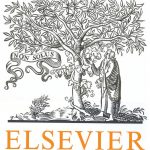
ترجمه مقاله پایداری توسعه شهری، تعدیل ساختار صنعتی و کارایی کاربری زمین
- مبلغ: ۹۱,۰۰۰ تومان
Abstract
Plate recognizer system is an important system. It can be used for automatic parking gate or automatic ticketing system. The purpose of this study is to determine the effectiveness of Genetic Algorithms (GA) in optimizing the number of hidden neurons, learning rate and momentum rate on Backpropagation Neural Network (BPNN) that is applied to the Automatic Plate Number Recognizer (APNR). Research done by building a GA optimized BPNN (GABPNN) and APNR system using image processing methods, including grayscale conversion, top-hat transformation, binary morphological, Otsu threshold and binary image projection. The tests conducted with backpropagation training and recognition test. The result shows that GA optimized backpropagation neural network requires 2230 epochs in the training process to be convergent, which is 36.83% faster than non-optimal backpropagation neural network, while the accuracy is 1,35% better than non-optimized backpropagation neural network.
1. Introduction
In the recent years, automated systems have become an integral part of daily tasks that only a human can do before. Automated systems are meant to help human to do task that involves knowledge, reasoning and experience. The integral part of an automated system is artificial intelligence and one of the application of artificial intelligence in automated system is Optical Character Recognition (OCR). OCR let a computer recognize character through visual interpretation and recognize character automatically without help from human. There are several algorithms that we can use to create OCR system, such as template matching, support vector machine (SVM), hidden markov model, hausdorff distance and artificial neural network. Artificial neural network is the most popular algorithm that has been used by researcher to solve pattern recognition problems1 . Artificial neural network can be used to solve many problems and it can be trained over time to gain its knowledge or to enhance its accuracy in recognizing patterns.
سیستم تشخیص پلاک خودرو سیستم مهمی است. این سیستم به منظور گیت پارک خودکار یا سیستم بلیت زنی خودکار قابل استفاده است. هدف این مطالعه تعیین اثربخشی الگوریتم های ژنتیک (GA) در بهینه سازی تعداد نرون های پنهان، نرخ یادگیری و نرخ حرکت در شبکه عصبی بازگشت به عقب (BPNN) است که به تشخیص دهنده خودکار شماره پلاک (APNR) اعمال می شود. این تحقیق با ساخت سیستم BPNN بهینه شده توسط الگوریتم ژنتیک (GABPNN) و APNR با استفاده از روش های پردازش تصویر شامل تبدیل grayscale، تبدیل top-hat، ریخت شناسی باینری، آستانه Otsu و نگاشت تصویر باینری انجام شده است. آزمایش ها با استفاده از آموزش بازگشت به عقب و تست تشخیص انجام شده است. نتایج نشان می دهد که شبکه عصبی بازگشت به عقب بهینه شده توسط الگوریتم ژنتیک نیازمند 2230 دوره زمانی در فرآیند آموزش به منظور همگرایی است به طوری که 36.83 درصد سریع تر از شبکه عصبی بازگشت به عقب بهینه است، در حالی که دقت آن 1.35 درصد بهتر از شبکه عصبی بازگشت به عقب غیر بهینه است.
1. مقدمه
در سال های اخیر سیستم های خودکار به بخش جدانشدنی کارهای روزانه تبدیل شده است. سیستم های خودکار ابزاری برای کمک به انسان به منظور انجام کارهایی است که دربرگیرنده دانش، استدلال و تجربه است. بخش جدانشدنی یک سیستم خودکار، هوش مصنوعی است و یکی از کاربردهای هوش مصنوعی در سیستم های خودکار تشخیص نوری کاراکتر (OCR) است. OCR به یک رایانه این امکان را می دهد تا کاراکتر را از طریق تفسیر بصری تشخیص داده و کاراکتر را به طور خودکار بدون کمک انسان شناسایی کند. الگوریتم های متعددی وجود دارد که می توانیم از آن ها برای ایجاد سیستم OCR استفاده کنیم که شامل تطبیق الگو، ماشین بردار پشتیبان (SVM)، مدل مارکوف مخفی، فاصله هوزدروف و شبکه عصبی مصنوعی است. شبکه عصبی مصنوعی محبوب ترین الگوریتمی است که توسط محققان برای حل مسائل تشخیص الگو مورد استفاده قرار گرفته است. شبکه عصبی مصنوعی به منظور حل بسیاری از مسائل قابل استفاده است و با گذشت زمان برای دستیابی به دانش یا ارتقاء دقت در شناسایی الگوها قابل آموزش است.