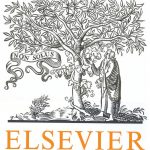
ترجمه مقاله نقش ضروری ارتباطات 6G با چشم انداز صنعت 4.0
- مبلغ: ۸۶,۰۰۰ تومان
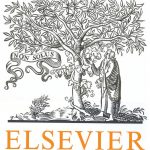
ترجمه مقاله پایداری توسعه شهری، تعدیل ساختار صنعتی و کارایی کاربری زمین
- مبلغ: ۹۱,۰۰۰ تومان
Abstract
Given the exponentially increasing volume of heterogenous medical cases, it is difficult to efficiently perform similarity-based reasoning (SBR) on a centralized machine. In this paper, we investigate how to perform SBR using MapReduce (SBRMR), which is an inference framework for data-intensive applications over clusters of computers. To combine the similarities from the individual machines, a mixed integer optimization problem is formulated to filter the priority reference cases. Besides, a resilient mapping mechanism is employed using a quadratic optimization model for weighting the attributes and making the neighborhoods in the same class compact, hence improving the inference capacity. Our experiments on classifying the medical cases demonstrate that SBRMR has approximately 4.1% improvement in classification accuracy over SBR, which suggests that SBRMR is an efficient and resilient similarity-based inference approach.
5. Conclusion
To design and implement similarity-based reasoning (SBR) in a parallel and distributed fashion, we develop the framework of SBR in MapReduce (SBRMR), performing medical case classification over clusters of machines efficiently. SBRMR first construct discriminative neighborhoods from each machine, then it combines all discriminative information in those neighborhoods to learn a single belief matrix. We formulate SBRMR as a mixed integer optimization problem and propose an efficient alternating strategy to filter the priority reference cases. Besides we design an effective mapping mechanism that exploits as a quadratic optimization model for weighting the attributes (or the distributed machines) and making the homogenous neighborhoods compact, and hence improve the inference capacity. Our experiments on classifying medical cases demonstrate that SBRMR has approximately 4.1% and 3.25% improvement in classification accuracy over SBR and Hz-KNNJ, which suggests that SBRMR is an efficient and resilient similarity-based inference approach.